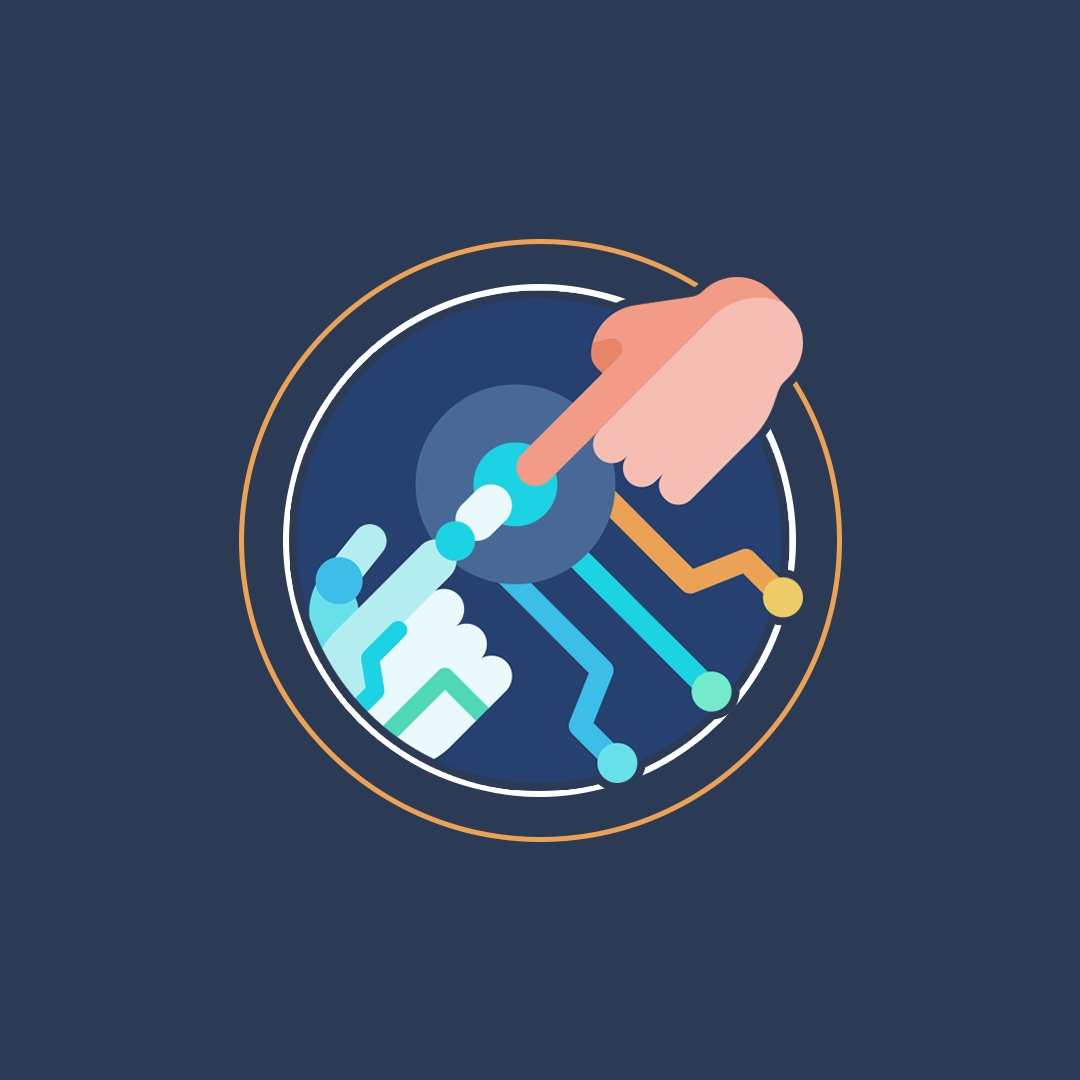
By Joseph Mercieca, CDP
Although Artificial Intelligence (AI) existed for some time, the AI race started when OpenAI released ChatGPT in November 2022. That moment marked a shift resulting in companies injecting AI into their products in addition to countless AI-first startup formations.
The fast adoption and consolidation of AI led to speculations on whether existing jobs notwithstanding design roles will be impacted. The World Economic Forum predicts that although AI will affect existing jobs, it will also create about 92 million new digital jobs by 2030.
Will AI replace or take over design or research?
Because AI is trained on vast amounts of data and programmed to learn independently, its capability to replicate tasks typically performed by humans could be numerous. As a result, an array of knowledge-related tasks like writing, coding, and computation can be handled by AI alone. Contrastingly, it is anticipated that novel jobs will be created without excluding the increasing demand for researchers, machine-learning (ML) engineers, and data scientists.
Will AI take over design roles? As things are right now, AI will not replace designers and related categories like UX, Interaction (IX), Product, Service Designers, and Design Strategists. Various reasons exist as exemplified below:
Human centricity
Design (e.g., UX, Service) is a discipline that belongs to Human-Computer Interaction (HCI). Cemented in human-centricity, the designer role calls for a deep understanding of human psychology including behaviorism and reasoning alongside the sanctity of the human soul (i.e., morality, mindfulness, ethics). In other words, what makes a human ‘human’?
For that matter, the presence of designers is essential to producing human-centered interfaces for AI-powered solutions, a task only humans can do. Consequently, a report by Future of Jobs, 2023 forecasts that businesses will prioritize UX and design in addition to big data from now till 2027.
Therefore, the demand for designers is expected to increase, most notably for specialized roles like Conversational designers, Chatbot designers, and AI Interface designers. While recalling conversations with my peers from the AI field, we anticipate witnessing the rise of the AIX Designer (defined as AI Experience Designer, or AI UX).
Ethics
The ethical aspect has always been under constant debate, hence the setup of regulations such as the European Artificial Intelligence Act. Although Artificial Intelligence simulates human intelligence to an extent, the risks cannot be ignored.
There are reliability issues as AI is prone to ‘hallucination’, which is when the AI model generates data that seems factual, yet it is not. Such eventuality could lead people to make wrong decisions based on incorrect information generated by the AI. This leads to accountability, such as who should be held responsible for the error.
Moreover, there are issues underlying transparency and discrimination. Imagine an applicant, such as for a mortgage gets rejected by an algorithm. That leads to the question concerning the validity of the rejection, hence the demand for informing users how the algorithm works especially if no human judgment is present behind such decisions.
Privacy and security concerns are debated, as to where and how the collected data is used, stored, and protected against malicious attacks. This is one of the reasons why ‘Sovereign AI’ (i.e., AI operated and managed by its native country) is nowadays becoming a hot topic.
Sustainability
To date, AI consumes water and energy massively. Thus, the question remains – is it sustainable to replace humans with machines?
How AI can help streamline design?
Subject to use cases, AI can play a role in the design process enhancing efficiency and creativity.
Examples include:
– Automation: Repetitive tasks can be streamlined via AI-driven automation allowing designers to focus more on strategic and creative aspects within the design process.
– Generative Design and Prototyping: Generative AI design tools can help designers with numerous concept designs and prototype variations thus facilitating quick feedback collection (stakeholders, users).
– Data and Predictive Analysis: AI helps analyze large data volumes to identify behavioral patterns and trends to make informed decisions based on accurate predictions relevant to product usage and habits.
– Auditing: AI-powered tools can audit newly designed concepts ensuring compliance with heuristic, accessibility, and other industry best practices.
– Personalization: AI algorithms can analyze customer data to help deliver personalized services based on individual preferences, behaviors, and needs leading to enhanced user satisfaction.
As a designer, how can I stay competitive?
Recalling the olden days, until its ubiquity to date, the prominence of the World Wide Web (WWW) introduced new jobs. Ever since, existing roles required skills relevant to current technologies. For instance, when smartphones were introduced, many companies sought designers with experience designing mobile applications. It is therefore expected that the demand for skills and/or experience relative to the AI domain will increase exponentially. Examples include, but are not limited to:
– Conversation Design
– Chatbot and Interactive Virtual Agent (IVA) design
– Prompt Engineering
– Information Architecture
– (Quantitative) Research
– Data annotation
Therefore, to retain a competitive advantage in this challenging market, I recommend brushing up your skills in, or learning (a few of) the following:
– Natural Language Processing (NLP) and Natural Language: Understanding NLU and NLP gives you an idea of how interactive AI applications work. Leveraging NLP and NLU helps craft interactive experiences for conversational interfaces.
– Information architecture (IA): IA plays a pivotal role in the AI domain because it helps assemble concepts (taxonomies, ontologies, vocabulary), their context, and relations systematically arranged in a way that can be retrieved and understood by people and machines alike especially when Large Language Models (LLMs) are involved. LLMs are AI models trained on vast data that help generate and understand human language.
– Data annotation: This involves the process of labeling, tagging, and transcribing a data set.
– Understanding data annotation would be helpful if you intend to collaborate with data scientists for your design project.
– Conversation design: Mastering conversation design helps craft engaging and effective conversation scripts while mapping out conversational journeys and flows to ensure quality human-to-machine interactions. Those versatile in storytelling, writing, and content design may find it easier to learn conversation design. Subsequently, you can gain a deeper understanding by building your chatbot. That way, you familiarize yourself with the process and requirements for crafting conversations.
– Prompt design/engineering: Generative AI relies on prompting. Knowing how to produce good prompting leads to quality output by AI interfaces. Prompt engineering requires a solid language command resulting in clear instructions that the AI interface can understand.
-Quantitative research: AI and ML are data and context-oriented. These require quantified data to learn, understand, and determine correlations and causations. Having practical knowledge of quantitative research is valuable particularly if you are designing an interface using custom data sets.
Optionally, exposure to linguistics, data science, and basic Python coding are pluses worth having. With the right demeanor and the will to learn from professionals in the AI field contribute to your competitiveness as a designer. In doing so, you will feel confident partnering up with data scientists and machine-learning engineers to tackle technical dependencies and requirements pertinent to the project you will be working on.
Conclusion
Hopefully, this article will serve as a stepping stone for you to gradually kick-start your learning journey towards designing for AI. That said, I highly recommend exploring and trying AI tools tailored for streamlining processes pertinent to research, collaboration, concept design, evaluation, and auditing. I have not included a recommendation list because tools keep prevailing online. Nevertheless, industry-standard tools by Miro, Canva, Adobe, and Figma have incorporated generative AI (GenAI) within their productivity tools. As well, don’t be afraid to get your hands dirty by browsing and trying chatbot builders. That way you will get a practical understanding about conversation design.